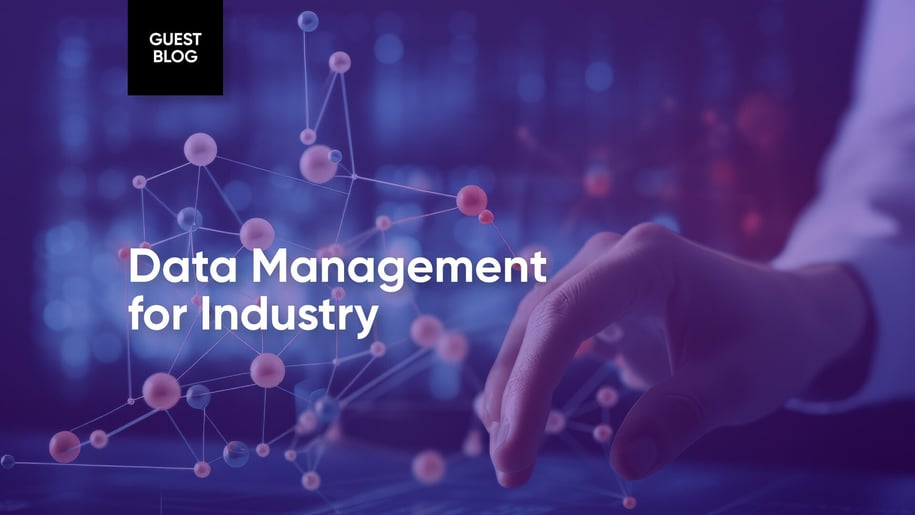
Find out how the right data management can lead to improvements along the entire value chain.
Change is both a challenge and an opportunity. When it comes to data management, the challenges and hurdles are often seen first. However, it is worth starting with the opportunities offered by well thought-out, modern data management. The aim is to capture data, store it appropriately, transfer it securely to any channel and ultimately not only improve quality, but also conserve the important resources of time and people. This blog is intended to help make this complex topic a little more transparent.
Consumer needs move into focus
In the consumer's perception, products are becoming increasingly interchangeable. What's more, competition is growing, but the potential customer base is not. As a result, most companies are confronted with the question: What makes customers buy my product and not the competitor's product? After many discount battles, the realization matures: the product itself and a low price alone are not enough.
This change may have come gradually, but it needs to be solved. What's more, customers today are better informed than ever before. There is maximum transparency about products, prices and quality. The customer experience is therefore becoming increasingly important. The focus on the customer, the ability to customize the customer experience, is becoming a unique selling point. One of the most important prerequisites for a good customer experience is an advantageous product presentation through better, usable and consistent information.
The customer expectation that goes beyond this is the "embedding" of products in a coordinated service structure. Companies that understand their customers' expectations and create and confirm positive experiences with their products and services stand out from other market players and are more commercially successful in the long term. This applies to the purchasing experience, the search for information, the service contact and every other interaction that a customer has with a company. Successful interaction between product management, marketing, sales and customer service shows how customer-centric organizations really are.
Data management for the industry
Many industrial companies have many products and are represented around the globe with these products. This means that the companies have achieved what they set out to do in the past: to be present on the global market. To achieve this, the companies have adapted their products for the target markets or even developed them specifically, including the necessary certifications and other accompanying measures. In addition, new structures in the form of dealer networks or subsidiaries have been set up to ensure successful sales.
Almost all companies share this success with a mountain of data and information that is growing faster than it can be kept in check or even reduced. Alongside products, markets and sales, the associated data management has gradually developed into a crucial component that determines success or failure.
Always close to the standard
Let's start with the benefits of data management for industrial companies. What does it actually involve and what determines its "industrial" character? In our case, data management does not refer to data for production, but to the management of product information, its enrichment and linking with other data. The aim is to create a database that is suitable for a wide range of uses in marketing, purchase and sales, customer loyalty and retail.
The focus on "industry" produces fewer specific requirements for data management than expected. This is a positive finding, as it allows us to stay closer to standards when it comes to solutions. Standard means a larger selection of software and more tried and tested processes. In other words: standard means fewer unpleasant surprises and more resources for the special features. The industry itself also provides us with a wealth of standards that we can use for our "Data management in the industry" project.
Single Source of Truth
In most cases, the management of data in companies has grown historically and the use of data rarely follows an overarching concept, but rather short-term needs. Instead, the required data is retrieved from somewhere, adapted for the respective purpose and stored where it is deemed useful. As a result, data can be found in different places in different forms throughout the company: Data freestyle instead of data management.
The first major milestone is therefore the creation of a common data source, the "single source of truth". This single source of truth (or "single spot of truth") is a central data platform within the company that can be accessed by all other systems. Ideally, this is done via a bidirectional connection so that data adjustments can be transferred from other systems. The single source of truth is one of the foundations for ensuring the consistency of data in companies.
Collecting data
In most cases, the basic data required for this can be found in the ERP system. In other words: the ERP system is the leading system in which articles are created and managed with the decisive information such as product name, article number, etc. Consequently, this raw data is transferred from the ERP system to the single source of truth. In addition, existing data sources are checked for usable data. These can be other systems - both internal and external - or individual documents such as Excel files. The data from these sources is also transferred to the single source of truth, where it is consolidated, i.e. merged and standardized.
This step follows a clear concept with defined data quality checks. As a result, the company receives the clean, centralized database required for all further steps.
Manage data
If you want to make large amounts of information more transparent and simplify processes, you have to look for commonalities between the individual parts. We are talking about data models that are able to describe products in a standardized way. Every physical product has characteristics such as dimensions and weight. These characteristics are summarized and described in a data model. If there are no or too few overlaps, another data model is created that represents the product group appropriately. This converts the product information into a defined, analyzable standard (see classification).
This work forms the basis for all further processes; the correct analysis and implementation is essential for overall success
Enriching data
After data classification and the creation of a central data point, the data is enriched with additional information. Data enrichment is the addition of further information to the bare product data. Every meaningful addition to this data increases its usability and thus makes it more valuable. The data can be enriched in different ways.
Manual enrichment
Employees/editors manually add further information to the data. Manual enrichment is often used in the marketing sector to provide products with suitable marketing texts. The enrichment itself depends on the purpose for which the enriched data is ultimately to be used. This type of processing should also follow defined processes that include quality checks and approvals.
Linking with other data
There is a lot of data within companies that is worth linking to the central data. Data from the ERP system, such as article numbers and EAN codes, but also from the DAM system, in which images, PDFs and other assets are managed, are of central importance. Data can also be linked within the single source of truth in order to relate products to each other. These relationships are important in order to realize cross-selling and up-selling in the store, for example. With data inheritance, properties and functions are inherited from main products to variant products for the benefit of efficient data management.
Integration of AI services
Artificial intelligence can be an invaluable aid when it comes to enriching data. AI models that are specialized in pattern recognition analyze images and thus help to tag or describe assets such as images. The AIs are served from the single source of truth and the results of the AIs flow back there. How automated or semi-automated the process is and how manual quality assurance is integrated is defined in the process.
Translation management
Internationality necessarily involves other languages. Translating content for the respective markets is therefore a familiar task, but with three specific challenges: Timeliness. Quality. Cost. The best way for companies to address each of these challenges is at the source, i.e. their single source of truth, and through a high automation. The easiest, quickest and most cost-effective way is for an AI such as DeepL to take over the translation tasks. Those who prefer native translations can integrate translation service providers into the processes.
Distributing data
Retailers, online stores, printed catalogs and assembly instructions all follow a common dictum: they want to be supplied with their specific data/content. The channels are served from one source, but only with the information that is relevant for the respective channel or medium. The same applies to the availability of data in CRM systems to support marketing automation. Advice or recommendation, automated or semi-automated - everything requires up-to-date product information with its numerous interrelationships.
Conclusion
Data management may work in the invisible, but the right data management is the linchpin for many success-critical processes in companies, including the integration of AI services. This makes data management one of the decisive components for digitalization and the future success of companies.