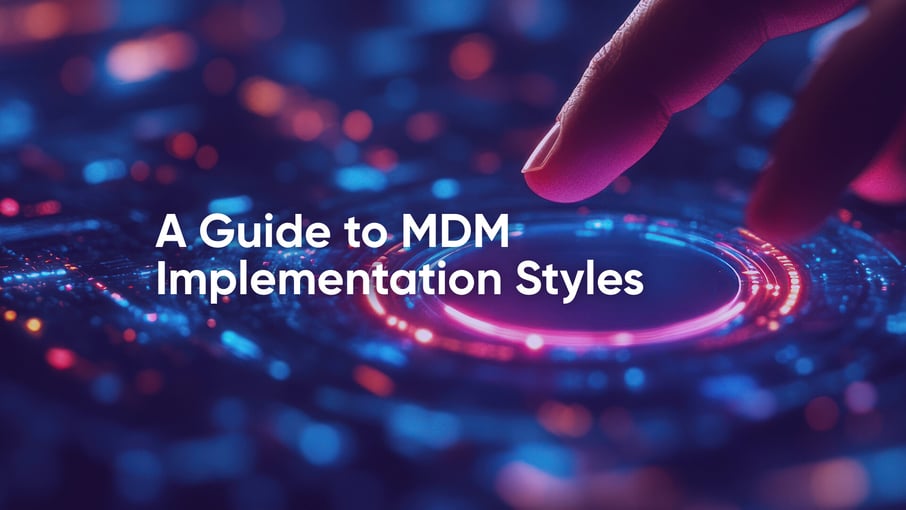
According to Gartner, “Master data management (MDM) is a technology-enabled discipline in which business and IT work together to ensure the uniformity, accuracy, stewardship, semantic consistency and accountability of the enterprise’s official shared master data assets...” This insight takes you through everything that exists about MDM. So, read on!
%20Beginner%E2%80%99s%20A%20to%20Z%20Guide_v1_R2_Final-01.png?width=790&height=445&name=Insight_What%20is%20Master%20Data%20Management%20(MDM)%20Beginner%E2%80%99s%20A%20to%20Z%20Guide_v1_R2_Final-01.png)
Introduction to Master Data Management (MDM)
When the decades-old practice of maintaining master data in various applications, technologies, and systems became unsustainable for enterprises, especially in the face of the growing volume and complexity of data, the discipline of master data management (MDM) came into existence. It required the IT and the business functions to work together and ensure uniform, accurate, and consistent master records were created and maintained through effective stewardship and management of the organization’s mutually recognized, shared data assets.
Mastering the Basics: An Overview of MDM
1. Definition and Core Concept of MDM:
Definition: Master data management (MDM) is a combination of sound data management practices encompassing all enterprise applications and platforms and involving key stakeholders, partners, and business clients. It includes consolidating, cleaning, standardizing, and augmenting corporate master data and synching it with business processes and analytical tools to implement necessary policies, services, and procedures across the enterprise infrastructure and to ease the capture and integration of data in a timely, consistent, and complete manner. The ultimate goal of an MDM system is to significantly improve operational efficiency, data reporting, and help businesses make smarter decisions.
2. Significance of MDM in Modern Business:
MDM emerges as an answer to enterprise data quality and consistency puzzle, as it creates a “golden record” of every piece of data added from numerous entry points. It is carried out by bringing together the business’s operational side, data warehousing, and business analytics. The data quality issue is eliminated by fixing the complexities across IT landscapes owing to the presence of a variety of applications, platforms, and systems. Various systems such as Enterprise Resource Planning (ERP), Customer Relationship Management (CRM), Order Management Systems (OMS), Supply Chain Management (SCM), etc., which have their own sets of data, are seamlessly integrated without jeopardizing operations, compromising analytics, and without costing enterprises dearly or affecting profit margins.
3. The Evolution of MDM
Businesses have realized that their survival is contingent on data-centric and agile architectures that are receptive to the unpredictable pace of change. For instance, active metadata (i.e., using open APIs to connect the tools in your data stack and transmit metadata in a two-way stream back and forth) and data fabric (where the architecture allows data pipelines and cloud environment to fully integrate via automation) are already transforming the MDM landscape. By leveraging active metadata analysis, an MDM program can detect, collect, and manage master data assets much more effectively. Additionally, Generative AI is starting to be seen as a driving force for discovering and curating master data. Besides, MDM vendors are moving to a cloud-based Software-as-a-Service (SaaS) model and are offering a comprehensive suite of managed services for MDM, significantly reducing the infrastructure-related barriers to adoption.
Overall, the MDM market is evolving, and MDM providers are going beyond and adding more capabilities to respond to the expanding data complexity.
What is Master Data?
Master data is the core information that fuels an organization’s analytical and transactional processes. It refers to the essential information (data of interest) pertaining to the entity being dealt with and runs across an organization’s digital ecosystem. Master data varies across industries. In the case of manufacturing, data of customers, products, materials, and suppliers is master data. For the medical industry, the data of patients, equipment, hospital supplies, regular medicines, etc., constitutes master data. For the retail sector, buyers’ names, contact details, buying history, loyalty program data, etc., are the master data. And in the insurance industry, it includes insured members’ data, policy details, product data, insurance claims, etc.
Types of Master Data:
One of the defining characteristics of master data is that it does not encompass the transaction details but defines transactional data such as the invoice number, invoice date, sub-total, etc. Master data can be segregated among a few key domains, such as:
- Customer Master Data: It contains information about customers with whom the enterprise conducts business, such as their names, addresses, contact details, and purchase history. This data includes customers, suppliers, partners, and employee information.
- Product Master Data: Product data pertains to the elements associated with the products and services offered; it includes data regarding products, assets, media, bills of materials, and equipment.
- Locations: Location includes the physical locations or the venues where enterprise work occurs, such as stores, agencies, facilities, franchises, and branches.
- Others: This domain contains all the remaining business-critical data that does not fall under the above three categories. This data can include accounts, certifications, financials, contracts, warranty details, policies, or other industry-related assets.
Challenges of Managing Master Data
Challenges emerge when different departments throughout the value chain, while making changes to the data, start creating their own version of it, begin storing it in silos, and reintroduce that modified data back into the system, leading to duplication, redundancies, ultimately hampering enterprise productivity and the overall output. In other words, when master data starts overlapping in various systems such as Engineering Document Management Systems (EDMS), Product Lifecycle Management (PLM), Manufacturing Execution Systems (MES), Asset Management Systems, CRMs, ERPs, and any other industry-specific systems, complications start showing up. Moreover, the incompatible legacy and homegrown systems working alongside various modern systems further contribute to the issue.
So Why is MDM Used?
A master data management (MDM) program sources data from multiple systems and offers a single view by consolidating all the data in a “golden” record. A master data management system promises authoritative, dependable data suitable to enterprises’ needs and objectives. For example, in the case of customer data, MDM standardizes all the customer data into a unique set of master data assets that can be used in all connected systems. This helps organizations remove duplicate data and eliminates redundancies and contradictions so that for everyone, be it operational staff, data analysts, decision-makers, or executives, there’s one and only one picture of every individual customer. An MDM program can be applied to customers, products, or any other kind of data. Some of the chief reasons why MDM is used are to:
- Improve Customer Satisfaction – Customer dissatisfaction can be a consequence of inconsistent, incomplete, low-quality data that can result in abandoned shopping carts, bad reviews, and severely damaged brand image.
- Boost Business Intelligence – Incorrect or incomplete data can never be the source of accurate analysis; it can not offer enterprises proper insight into market trends, customers’ intent, or how the data can be used beneficially.
- Get a 360-degree View of Data– Without a centralized repository, finding and knowing which data is correct across disparate locations, systems, applications, and other sources, enterprises can’t get a comprehensive view of the data, leading to unnecessary complications.
- Fix Unstructured Data Issues– Unstructured data doesn’t have an already defined schema or data model. It can be textual or non-textual but can be a cause of concern as it can lead to ambiguities.
Master Data Management Capabilities
- Data Cleansing and Validation: Data cleansing refers to spotting any discrepancies or inconsistencies in the data, understanding if they are sustainable or not, and fixing it to ensure data stays accurate before being ingested into the new platform. Data validation refers to verifying how accurate or complete the data migrated in the MDM system is.
- Data Mapping and Transformation: It refers to transforming the data in your existing system and loading it into the MDM system; this process is highly dependent on sound knowledge of data structure and relationships.
- Data Quality Management (DQM): There are different dimensions to DQM. One of the most important ones is defining the data quality rules from profiling to cleansing to completion and finally protecting the data. DQM also includes constant measuring and monitoring of the data quality and is quickly becoming a differentiator for MDM providers.
- Data Integration and Consolidation: Data scattered across geographies or acquired through mergers and acquisitions needs consolidation. Data integration with other enterprise systems, like ERPs, CRMs, etc., is required to ensure interoperability and smooth interaction between platforms and applications for seamless data exchange.
- Data Governance and Data Stewardship: Data Governance pertains to charting the data definitions, access rights, standards, and quality rules and executing those rules to enable proper data controls across the organization. Data stewardship supervises the enforcement of policies and guidelines by collaborating with business and IT functions.
Benefits of Master Data Management
i. Improved Operational Efficiency and Higher ROI
MDM helps enterprises to function with amplified acceleration and agility. It boosts the association between applications, systems, and people by integrating disconnected processes to drive performance, manage better workflows, promote collaboration, and reduce risks. It converts duplicate, unreliable, contradictory data into a single, authoritative form of truth—a golden data repository. Efficient master data management directly affects enterprise ROI, as all the data-oriented activities depend on the quality of relevant data and strong collaboration. Organizations can support vendors and employees with streamlined processes, saving time and resources; marketing and sales teams can target customers efficiently by adjusting to their expectations, thereby maximizing the ROI.
ii. Excellent Customer Experience (CX)
MDM aids the prevention of disparate and segmented product lines in companies across geographies, preventing scattered product data from reaching multiple customer touchpoints and channels. Hence, enterprises eliminate data irregularities and duplication, becoming the bedrock of delivering personalized and engaging product messaging to attract customers. It genuinely improves the ability to acquire new consumers, build and nurture existing customer relationships, further advocacy, and preserve enterprise reputation. By maintaining the highest quality of data, right from the first interaction with customers to purchase, shipping, delivery, and feedback, MDM makes sure customer experiences remain impeccable.
iii. Faster time-to-market
With accurate, reliable, streamlined data, products and services can be delivered to the market smoothly and quickly, especially across retail, CPG, manufacturing, or the automotive industry. MDM guarantees that data can be updated and maintained, accelerating productivity, data governance and compliance policies, streamlining security protocols, and various internal processes. All of this dramatically reduces the time-to-market. Moreover, MDM lets enterprises glean insights and underpins faster people and process coordination, which aids in introducing changes and implementing decisions into the system much more swiftly. MDM impacts the time-to-market not just via enhanced data quality but by quickening enterprise adaptability.
iv. Enhanced Decision-making
With a 360-degree view of data across the entire ecosystem, decision-makers can quickly analyze any glitches or effectiveness of the processes and draw the right inferences to optimize productivity, performance, vendor collaboration, and customer service. The improvement in speed, timing, relevance, and analysis rendered by an advanced MDM program significantly enhances organizational decision-making capability. With data being trustworthy, the decisions become trustworthy, too. MDM also enables better monitoring and reporting to drive optimal risk assessment and opportunities, further influencing decisions. Additionally, through superior technologies like data fabric and active metadata, MDM’s scope can be broadened, and previously unconnected data can be brought into the fold.
v. Reduced Risks
MDM allows for comprehensive risk reduction, including:
- Regulatory risks – Offering complete or correct views of customer communications and minimizing counterparty risks concerning financial establishments defaulting on contractual obligations.
- Operational risks – Decreased supply chain risks emanating from poor visibility, e.g., observing the performance of specific materials within a manufacturing process.
- Security or infrastructure risks – These are minimized as the MDM program offers a comprehensive view of all the data and physical assets.
- Data and governance risks – They decrease due to the accuracy of gathering intelligence about the location or usage of specific data assets.
What is Master Data Governance?
1. Essential Features of Master Data Governance:
- Identification of data resources that need to be managed.
- Delegation of responsibility for data resources.
- Communication about how the governance structure is to be laid out and how the processes will be followed.
- Allocating decision-making rights in relation to accessing common data resources.
- Handover of roles and responsibilities to distinct areas of any implementation, including policies and processes for all common data resources.
2. Who Are the Stakeholders in Master Data Governance?
- Executive Level: The executive layer, or the uppermost layer, involves the top-level management, who are experts in developing policies and strategies; they’re mostly the C-Suite executives who supervise the governance of data from above.
- Strategic Level: This comprises the individuals who set the policies in practice and offer a pre-meditated path to data. They are also liable for the resolution of escalated issues from a strategic perspective.
- Tactical Level: These individuals carry out tactical-level data troubleshooting. They outline, monitor, and report on tactical activities and metrics. They also handle the data quality and integrity across functional areas, lines of businesses, and geographies.
- Operational Level: This level comprises people accountable for complying with data standards, effective data production to resolve data-linked issues, managing operational data governance metrics, and enforcing data standards. These people are the data custodians, data liaison officers, application data architects, and application owners.
Industries Where MDM is Used
Retail
As the retail industry turns towards a customer-driven, personalization-laden, unified view of authoritative data, they need faster data onboarding, constant agility, streamlined processes, automation, flexibility, and operational efficiency. MDM offers pre-configured retail-centric elements, dashboards, business rules, data models, and user interfaces needed for constant innovation, speed, engagement, and digital transformation to drive ROI and quick time-to-value.
CPG
With manufacturing and supply chains becoming ever more data-intensive, partly due to evolving customer buying behavior and partly due to increasing touchpoints, CPG businesses need better insights and a single view of the entire ecosystem. MDM helps them gain clarity through a holistic view of the business ecosystem, including distributors, wholesalers, and other network partners. It improves reporting across the value chain, imparting CPG brands the competitive edge they seek.
Manufacturing
Improvement in compliance, data automation, and workflows are paramount for manufacturers to integrate information from various systems in order to keep up with suppliers, distributors, and end-users. MDM solution standardizes data, enabling products to get to the market faster, with improved data governance and bi-directional information sharing with recipients. MDM allows product data syndication to meet the needs of multiple channels that require data in various structures and formats.
Distribution
Distributors entrusted with managing millions of SKU (stock-keeping units) need real-time insights into business-critical data, a complete view of product flows, inventory in stores, sound compliance, and to calculate accurate ROI. MDM solutions through consolidated multi-domain data, advanced inventory management, streamlined flow of information, and enhanced workflow and communication can forge better distributor links with retailers and wholesalers via on-time delivery and correct information.
Automotive
To keep up with technology and industry transformations, market pressures, customer preferences, and changing regulatory and compliance requirements, automotive companies have to deploy MDM \to manage the complex master data flow involving manufacturers, suppliers, distributors, car dealers, and after-sales partners. Effective MDM implementation can centralize data, streamline operational processes, and integrate various systems related to supply chain, sales, retail, and customers.
What is Single Domain and Multi Domain MDM?
Single Domain MDM
When MDM is implemented for a particular data domain, it is referred to as single-domain MDM.
For example, MDM for products, aka product master data management, focuses solely on enterprises managing master data related to their products. It consolidates all the product data in a master repository, creating a single version of truth by managing and sharing it across the entire enterprise ecosystem.
Product MDM ensures that data accuracy, transparency, and consistency is maintained across the entire value chain. It streamlines product data complexities and creates competitive advantages for businesses. It helps share the right product data at the right time with the right individuals.
Multi-Domain MDM
As enterprises’ interest grows in managing more than one data domain, such as customer data, digital assets, channel/partner and vendor/supplier data, and geospatial and hierarchical data, it means they need a multi-domain MDM.
A multi-domain, multi-vector MDM enables digital businesses to collect data in several single domains while connecting the data in those domains.
Multi-domain MDM solutions assist enterprises in exploring data associations, striking connections, defining entities, and generating newer possibilities to grow their business.
The progression towards a multi-domain MDM has emerged as a natural step for enterprises.
How to Choose the Right MDM Platform
It is essential for businesses to first develop and formalize a vision of how an MDM solution will support their enterprise and help them achieve long-term value.
Before anything, top management executives must nurture a new mindset regarding data usage and management, where data housed in siloed applications, individual systems, and incompatible legacy software is discouraged and gradually rejected. High integrity and high confidence in master data are at the heart of every MDM strategy.
Choosing the right MDM platform is critical for organizations looking to manage and ensure the quality of their master data. Here are certain vital factors to consider when selecting an MDM platform:
- Data Integration and Interoperability: Assess the platform’s ability to integrate with your organization’s various data sources, applications, and systems. Consider support for different data integration methods, such as batch processing, real-time integration, and data virtualization.
- Scalability and Performance: Ensure that the MDM platform can scale to accommodate the volume of data and the number of entities within your organization. Evaluate the platform’s performance in handling large datasets and complex data relationships.
- Flexibility and Customization: Look for a platform that allows flexibility in data model customization to meet your organization’s unique requirements. Assess the platform’s ability to adapt to changing business needs and evolving data models.
- Data Security and Compliance: Ensure the MDM platform complies with data security standards and regulations relevant to your industry. Evaluate features such as role-based access controls, encryption, and audit trails to secure sensitive master data.
- Data Governance and Quality: Evaluate the platform’s data governance capabilities, including data quality management, data profiling, and data cleansing. Look for features that allow for establishing and enforcing data standards and policies.
- Workflow and Business Process Integration: Consider how the MDM platform supports workflow automation and integrates with your organization’s business processes. Look for features that enable the orchestration of data-related processes, such as data validation, approval workflows, and data stewardship.
- Data Mastering and Matching Algorithms: Evaluate the platform’s data mastering capabilities, including identifying and managing duplicate records through sophisticated matching algorithms. Look for tools that help resolve data conflicts and ensure a single, accurate version of master data.
- Metadata Management: Assess the MDM platform’s metadata management capabilities, which include the documentation and management of data definitions, relationships, and lineage.
- Usability and User Interface: Consider the MDM platform’s ease of use and user interface, as they can impact user adoption and the efficiency of data management tasks.
- Vendor Support and Community: Evaluate the reputation of the MDM platform vendor, their support services, and the availability of a user community or forums for knowledge sharing and troubleshooting.
Enterprises can choose an MDM platform that aligns with their data management needs and objectives by carefully considering these factors.
MDM Use Cases
Global tech titan tackles 20+ million SKUs across 30 countries
The enormous technology conglomerate, spanning 64 countries and 6 continents, urgently required a versatile, resilient, and scalable product master data management system to oversee and centralize over 20 million product SKUs across 30 countries. Pimcore seamlessly accomplished this task by replacing their existing product information (PI) system with product MDM.
Pimcore initiated the process by amalgamating data from various sources like ERPs, internal databases, vendors, and other critical repositories into a unified portal. This resulted in a comprehensive and editable single view of product data, alongside the effective management and consolidation of digital assets using Pimcore DAM.
Consequently, Pimcore successfully unified 1500 categories and 60,000 product attributes, encompassing nearly 1.7 million drop-down values. This led to a substantial reduction in data processing time, ensuring the accuracy and currency of the data. The cohesive presentation of product information significantly improved user accessibility.
Additionally, Pimcore implemented a standardized data model to convert product master data from diverse sources, consolidating it based on rules relevant to their customers or marketplaces. Simultaneously, Pimcore empowered vendors by enabling them to upload and enhance product data within the system. This not only addressed the conglomerate's current product master data management requirements but also positioned them effectively for future needs.
Global automotive giant managed 45K SKUs in 45 languages and 50 countries
The organization sought a centralized Product MDM system to serve as an authoritative source by consolidating and overseeing approximately 45,000 product SKUs, maintaining the information in over 40 languages, and facilitating distribution in more than 50 countries worldwide. Previously, product information was scattered across systems like SAP and MS Excel, with communication occurring through emails. This decentralized approach led to data fragmentation among multiple users and disparate systems, including manual sharing of digital assets.
Pimcore implemented a robust Product MDM and Digital Asset Management (DAM) to centralize, control, and manage both product information and digital assets. Tailored workflows were established, and digital assets were centralized to enable the dissemination of data in multiple languages on an international scale.
Consequently, a printable price catalog, containing details on over 5,000 products across 300+ pages, was developed for distribution in more than 30 markets. The platform also facilitated the management of marketing data, automated quality checks through an approval process, and ensured the customized export of product data based on intricate transformation rules specific to consumers. Pimcore's contributions led to a reduction in data retrieval time, enhanced data usability and visibility, and simplified the global management of assets. The positive outcomes significantly improved customer experience and streamlined operational processes for the business.
Future Trends in Master Data Management
Use of Artificial Intelligence (AI) and Machine Learning (ML)
AI and ML are already assisting in the simplification of MDM. Apart from improving data quality, automating data governance, and facilitating real-time data analysis, they help in suggesting product categorization for quicker new product introductions (NPIs), facilitating data stewardship via advanced automation and intelligence, and automating data modeling and mapping recommendations. Generative AI is already coming up as a catalyst to speed up the discovery and curation of master data. By and large, automation and AI can help organizations quickly perform repetitive, monotonous tasks and speed up the management of ever-expanding volume and variety of data without bringing more resources on board.
Cloud-Based MDM Solutions
Enterprises are adopting a cloud-first mindset to steer their MDM solution towards better productivity, cost reduction, and higher agility. Cloud MDM simplify the migration of applications and the consolidation and integration of databases. The microservices-based modern architecture, scalability, and flexibility that come with pay-as-you-go pricing make it particularly viable for businesses. Besides, the ease of use for data stewards, business users, and IT administrators and the quality of being accessed anywhere, anytime makes it even more helpful. Most importantly, cloud-based MDM has reduced the entry barrier for MDM via subscription-based licensing model, significantly improving the time-to-value for enterprises.
Context-driven MDM
MDM’s contextual characteristics are the best way to follow the iterative and agile technique compared to the traditional methods. Contextual MDM is increasingly used by organizations that want to rely on data-centric decision-making and have a strategic focus. Many data management technologies come together to support contextual MDM to build the capabilities for enriching, connecting, and democratizing data with agility and quickness needed in an ever-evolving business environment. Contextual MDM will continue to provide businesses an edge in delivering superior CX by connecting transactions and interactions with customers; it is especially useful in managing customer master data.